History of Artificial Intelligence
Information Technology
15 December 2020
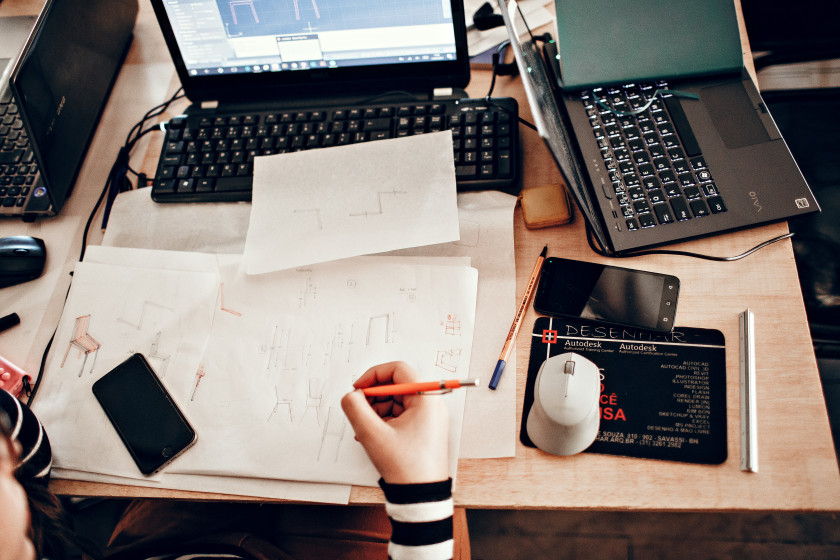
At times, science fiction novels and films have presented an alarmist view of artificial intelligence (AI), featuring scenarios in which machines try to destroy us and take over the world. Fortunately, Dr Henk Roodt from Capable NZ is here to assure us of the strengths and limitations of AI – which he prefers to call ‘computational intelligence’.
Dr Roodt is a senior IT and postgraduate facilitator at Otago Polytechnic’s Capable NZ. He notes that the first AI technology dates back to 19th century Europe. “The first machines were gear-driven computing devices designed for playing chess with,” he says. “Chess has long been considered a game of deep thought and intelligence, and the development of these chess-playing devices has continued ever since. Some of the greatest chess masters of our time have played these machines, and a computer won for the first time only a decade or so ago.”
This is because, prior to the 1960s, these machines would have to evaluate all possible moves during the game before they took any move. To do this efficiently would require a massive search function driven by immense computing power. Since the 60s, new algorithms have assisted the computers, allowing them to perform this task better than before. The machines have been able to “learn” from the decisions of the humans they have played, factoring these in when taking their own actions.
If this sounds worryingly like human intelligence, Dr Roodt assures us it isn’t.
“Intelligence goes beyond algorithmic learning,” he says. “This is why I use the term computational intelligence (CI). It is possible to mimic intelligence using computation, but it remains computing and we humans are always in control of the machines. And humans know how to deal with new and uncertain events under pressure.”
Dr Roodt has had many conversations over the years that frame CI as a threat. “There’s this idea that the machines could get out of hand. They have astonishing capabilities, but in the end, they are driven by the algorithms and commands that we programme them with. This is a far better frame of reference – these devices should be thought of as useful, rather than something to fear.”
He acknowledges that in the hands of people with ill intentions, machines can do terrible things.
“This is true throughout history – humans have created many harmful machines. Certainly, there is a need for people to be responsible in their work with CI.”
Dr Roodt says, overwhelmingly, the technology presents many exciting opportunities.
Approaches in design
Artificial neural networks first emerged as humans studied the brain – the nerve endings, synapses and chemical construction of human neurons. We then sought to have computers imitate these human neural processes.
“As in biology, this technology sent an electrical pulse along a ‘nerve’, triggering a chemical reaction that sent signals to other ‘nerves’. However, if the electrical pulse was too weak, the chemical process would not occur and nothing would happen. This is what we call a non-linear response – where input does not always equal output.”
The concept was developed and shown to work in principle by Bernard Widrow under a US Naval Research contract in 1960. He developed various combinations of the device, but ultimately, the work was too complicated to be solved with the technology available at the time. The concept of a resistor with a logical memory is still under development in nanotechnology labs and holds great promise for large parallel computers of the future.
“The next development was rule-based, like the original chess machines,” says Dr Roodt. “A tree of decision choices drove output. Computing engines were cheaper, had more memory and were easier to programme. This was when algorithm development started.”
A breakthrough in programming computational intelligence came with feed forward neural networks. These involved entering a pattern and the computing machine could adjust internal responses or weights to approximate outcomes comparing them to sets of exemplars, and as a result develop recognition and prediction of previously ’unseen’ inputs.
“The machine was learning patterns using smart algorithms to fine tune the mathematical neural networks,” says Dr Roodt. “It could also make adjustments based on outcome, updating patterns when it made incorrect decisions – so, over time, these machines networks could become ‘smarter’.”
To develop this kind of AI further, the machines would need the ability to identify and extract the most important and distinguishing features of any pattern. In the 80s, learning algorithms were working well, but automatic feature extraction remained problematic. “Many complicated algorithms were created to try and solve this issue, but computing power was limited and prevented success,’ says Dr Roodt.
Eventually, around 2010, certain discoveries around effective feature extraction processes emerged and were able to feed into neural networks. There had been massive computing power advancements, and algorithms were slick and readily available through access to open source software and computing languages.
Another advancement came by way of adaptive agents. “This is a simple computational construct meaning that a group of machines or agents can collectively work together using smart collaborative processing,” says Dr Roodt. “This technology pairs nicely with neural networks, creating the technology to control self-drive cars, for example.”
Genetic algorithms tap into the way our genes adapt and ‘learn’ over time, establishing very similar processes in the development of CI technology. “We now have very smart genetic algorithms that can evolve in response to various situations, and we can stimulate these in certain ways to drive effects.”
These astonishing technological advances have required massive increases in computing power every decade over the past 70 years.
Dr Roodt says now that we can combine all these forms of CI, they assist and augment our lives and our decision-making and in everyday computing. “A good example is the built-in neural network chips in the new Apple Silicon computing hardware for Apple Mac, iPhone and iPad, released this year. CI has gone commercial!”
These advances can be as helpful to us as corrective lenses or hearing aids, he says. “They can be found in a range of cutting-edge technologies like imaging machines in health care, which can detect precursors to illness through image processing much faster and earlier than human analysis could. Likewise in aviation – adaptive software uses CI technology to help keep planes in the air. Your mobile phone’s thumb print and facial recognition abilities, or your smart watch’s heartbeat monitoring, is driven by AI through pattern recognition. This technology is helping us to make better sense of the world and to deal better with the complex decisions we make every day.”
Capable NZ offers project-based learning, work-based learning and open-ended study plans in IT at undergraduate and postgraduate levels. Capable NZ also provides professional development training within businesses and organisations on the latest in IT innovations and practices. Contact Capable NZ to learn more.